- Onyx 3 2 2 – Maintenance And Optimization Tool Set
- Onyx 3 2 2 – Maintenance And Optimization Tools
- Onyx 3 2 2 – Maintenance And Optimization Tool Software
- Onyx 3 4 2 – Maintenance And Optimization Tool Software Download; OnyX is a maintenance, optimization, and personalization utility for Mac OS X. October 22, 2018. Dual Monitor Tools is a software package for Windows users with dual or multiple monitor setups. Titanium Software. This website provides operating system utilities for macOS.
- OnyX 3.2.8 – Maintenance and optimization tool. OnyX is a multifunction utility that you can use to verify the startup disk and the structure of its system.
OnyX is one of the best free utilities for maintenance, personalization and optimization of your MacOS.Not only that it is capable to run countless miscellaneous tasks of system maintenance, but it enables you to easily find and tweak many hidden parameters of Dock, Dashboard, Safari, Expose, Disk Utility and Finder.
Offshore Wind Farms are costly assets, not only in the aspects of design and construction, but also in those of operation and maintenance. Their remote location, along with weather constraints, harsh marine environment, and lack of adapted access equipment, make their maintenance complicated and expensive due to high repair and transportation costs and painful production losses generated by high downtime.
Onyx 3 2 2 – Maintenance And Optimization Tool Set
Generally, offshore wind technology is in a stage where its economic viability has yet to be fully proven, and numerous projects are largely supported by governmental subsidies [1]. This lack of maturity is evident, for example, in the numerous reliability issues related to wind turbine components.
Both the inherent aspects of the offshore wind industry and the reliability problems it faces contribute to high Operation and Maintenance (O&M) costs, which eventually hinder its competitiveness compared to other sources of energy. It is commonly assumed that up to 25 % of the cost of energy produced by offshore wind turbines is generated by O&M activities – twice as much as with onshore installations [2].
This article describes a methodology for optimizing the maintenance strategy of offshore wind farms, in order to minimize lifecycle costs, and maximize availability and performance. apmOptimizer, developed by BQR Reliability Engineering, [3] is an advanced software tool used for modelling, reliability analysis, cost and performance estimations, as well as maintenance optimization.
For the purpose of the demonstration, we have created a case study of a typical wind farm, including a detailed model covering numerous operational, cost and maintenance parameters. The apmOptimizer optimization modules are then implemented in order to optimize the preventive maintenance, periodical inspections, condition-based maintenance, and spare part levels. Starting from a baseline scenario, several optimization steps are investigated, following a typical RAM/LCC analysis assessment procedure: Operational Availability, Operational Downtime, Lifecycle O&M Costs and produced energy are used as the main metrics. The optimal maintenance strategy is obtained from an exact optimization process and not from Monte Carlo simulations, which can provide only an approximated assessment for a user-inputted strategy.
The case study clearly shows that by following several optimization steps, the wind farm's performance can be significantly increased, allowing the produced energy's cost to become substantially more competitive. The impact of modelling parameters such as failure distribution and degradation process is also investigated.
1. Background on the apmOptimizer
apmOptimizer is a Modelling, Analysis and Optimization tool designed to bring asset maintenance to an optimal state, maximize availability and minimize Cost of Ownership over lifetime. Optimization algorithms are implemented to identify the optimal solution among all possible outcomes, according to the problem constraints and targets. apmOptimizer is not based on the Monte Carlo method whose result accuracy is based upon the number of calculated simulations and is dependent on user's best guess, but, instead, performs exact analytical computations from the input data, including failure rate distribution and reliability model (serial, parallel, standby redundancy, K out of N). The optimization process itself is not user-driven, since the space of possible solutions is based on these exact computations. This guarantees that even non-evident solutions can be identified.
In this case study, apmOptimizer is used to build a model of a typical wind farm and calculate its Reliability Availability Maintainability (RAM) parameters, as well as Lifecycle Costs (LCC). The Energetic Performance of the wind farm is also computed and the cost of energy extracted. The apmOptimizer model can integrate the most specific features of a wind farm and of its maintenance operations: Accessibility limitations, weather variability, high cost of downtime, planned and unplanned maintenance, etc. Although this is not done in the present case study, operational data from an actual wind farm (maintenance records, log book, sensor readings) can also be used for a better assessment of failure distribution.
After defining and characterizing a baseline model, several scenarios have been created, that successively investigate the impact of preventive inspections, preventive replacements, and inventory level. Each of these scenarios is provided to apmOptimzer for optimization and RAM/LCC and Performance Metrics are compared.
2. Description of the Model
This case study's scope covers only a brief review of the main elements, and not a complete description of the input parameters. The authors would like to highlight the fact that the data used in this work was obtained from publicly available sources and unavailable data was assessed by engineering judgment.
2.1 Wind farm elements
The considered system is a 240MW wind farm, composed of 80 generic wind turbines, each with a rated power of 3MW, one offshore substation and submarine cables. Turbines are grouped in 8 clusters (radials), each containing 10 WT and cables. The farm is located at a medium distance of 30 km from shore. The wind farm (WF) operates on a 24/7 basis, with an expected lifetime of 20 years. Since this case study is focused on evaluating the costs related to O&M activities only, it is assumed that the wind farm is completely operational from year 1 and that commissioning and decommissioning phases are not considered for cost analysis.
It is assumed that each 3MW wind turbine is pitch-regulated, with variable speed, with gearbox installed, and is modeled by a breakdown structure – see Fig.1, adapted from the Reliawind project [4]. For the sake of simplicity, the breakdown tree is limited to assembly level, and sub-assemblies and components are not modeled.
2.2 Failure and reliability parameters
The global wind turbine failure rate was assumed to be 3.8 failures/turbine/year, based on an initial onshore data.
Fig. 1: Wind Farm Breakdown (screenshot)
Value of 1.5 and conversion coefficientsK1=1.75 and K2=1.447 for environmental stress and near shore location respectively [5]. The components contribution to total wind turbine failure rate was then obtained from [6], where exponential distribution is assumed.
In accordance with [7] approach, we have defined several generic failure modes (FM) for each element of the wind turbine and for each failure mode a failure ratio (FR). For example, the gearbox (GB) has 3 failure modes defined as shown in Table 1:
Name | FR | Detectability | Type | Degradation to failure, hrs | deviation |
GB-1 | 0.45 | Evident | – | ||
GB-2 | 0.45 | Hidden | Gradual | 20000 | +/-50% |
GB-3 | 0.1 | Evident | – |
Table 1: Failure modes definition for the gearbox
The failure modes, having some inspection tasks, are defined as hidden (others are evident failure modes), meaning the failure cannot be detected until an inspection is performed. Failures detected by condition monitoring equipment are considered to be evident. Additionally, the degradation process of hidden failure modes is modeled by defining a 'mean time from degradation start to failure', as well as a 'deviation' parameter representing the uncertainty of the degradation process.
2.3 Maintenance tasks and resources
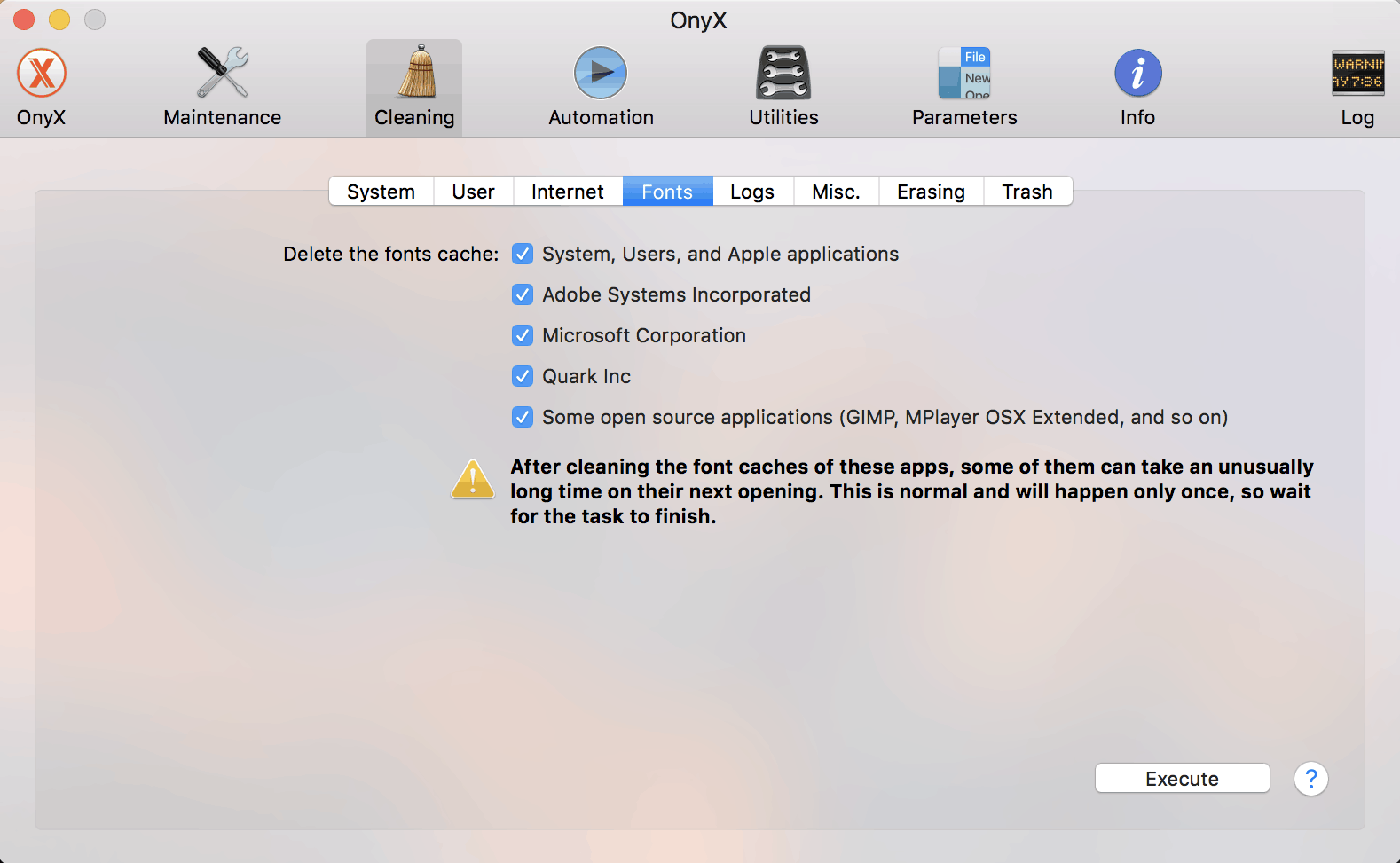
The failure modes are assigned some maintenance tasks and maintenance resources required to restore the equipment in accordance with [7].
The costs of personnel, support equipment, materials and other resources are defined in libraries and allocated to maintenance tasks. The wind farm is maintained by 20 technicians working 12 hours per day, 350 days a year at a cost of 70Є per hour. Operations personnel (stationed in a control room) is composed of 3 teams of 3 operators (8-hour shifts) at a cost of 50 Euros per hour.

The failure modes are assigned some maintenance tasks and maintenance resources required to restore the equipment in accordance with [7].
The costs of personnel, support equipment, materials and other resources are defined in libraries and allocated to maintenance tasks. The wind farm is maintained by 20 technicians working 12 hours per day, 350 days a year at a cost of 70Є per hour. Operations personnel (stationed in a control room) is composed of 3 teams of 3 operators (8-hour shifts) at a cost of 50 Euros per hour.
2.4 Maintenance locations
Most of the maintenance tasks are performed on-site and only the heaviest repair tasks like blade or gearbox repair are performed in an on-shore maintenance facility. Spare parts are also stored in this facility, where they arrive from different suppliers or after repair. Spare parts procurement takes place on-demand and a time delay that accounts for production and/or administrative delays is defined for every replaceable item. For example, the blades procurement delay is 6 days. The maintenance locations and transportation between them are defined in the apmOptimizer through the maintenance map shown in Fig.2.
Fig. 2: Maintenance Synoptic Map (screenshot)
Several access vessels are defined between the wind farm and the maintenance facility:
- Cable laying vessel – used for cables
- Jack-up vessel – used for large parts
- Workboat access vessel – used for transporting personnel
- Diving support vessel – used for inspecting foundations
Wind turbines are equipped with an internal crane that can handle small parts, while larger parts require use of the Jack-up vessel.
Supply of spare parts to the maintenance facility is performed by a standard truck with an average transportation time of 20 hours, except for the blades, where a special truck is used, with an average transportation time of 120 hours.
2.5 Environmental parameters
The wind variability is the most significant factor in the energy production. Its variation is mostly seasonal and widely studied in professional literature [8]. In the present case study, wind speed variability is accounted for on an annual basis within the energy production module, by assuming a wind speed density function of Weibull type, with scale parameter A=8.09, shape parameter k=1.96 [9], and a rated power output curve of the Vestas V90 3MW WT, as shown in Fig.3. By combining this data with the reliability metrics of the wind farm components, the total produced energy at wind farm level, as well as its cost, can be extracted.
Fig. 3: Assumed WT Power Curve and wind speed distribution
An Initial performance computation yields an ideal (no failures) annual energy production of 960096 MWh.
The second major impact of the wind variability is its delaying of maintenance operations. This is accounted for in our study through additional mean transportation time delays incurred by the transportation vessels.
3. Baseline Scenario
3.1 Initial maintenance strategy
Autocad 2016 4 download free. The basic assumptions of the initial maintenance strategy are described hereunder:
- Inspection of all components is performed once a year for all components.
- When an inspected item's degradation is too severe or has failed, a spare part is ordered from stock.
- If a spare item is available in stock, it is sent to a wind farm with required maintenance resources by using the appropriate vessel; if not, it is ordered from the supplier.
- Stock delay due to administrative constraints is assumed to be 24 hrs.
- Suppliers delays are item dependent (several days in all cases).
- Transportation delays incorporate: Mobilization time, transportation time, positioning time, and an assumed weather variability delay.
- When a spare part arrives at the wind farm, the item is removed and replaced by the new one
- Failed item is taken back onshore for repair at repair facility according to defined tasks. A repair delay (for administrative procedures) of 2 days is assumed in addition to repair time.
- Repaired item is made available in stock.
- Some items have repair condemnation, meaning they can be repaired only a limited number of times, before they are disposed of. In this case, a new spare part is ordered.
3.2 Analysis Results
Computations are performed to extract the main characteristics of the wind turbine: Reliability metrics, performance metrics and cost metrics. Figs. 4 and 5 show the contribution of each component to the total failure rate and to the total computed downtime.
|
The pitch and yaw systems are clearly the main contributors to the downtime, along with the generator and gearbox. This is mostly due to a relatively high failure rate combined with a high turnaround time: When a part is in need of replacement, a long period of time is required for the the jack-up vessel to be available and reach the wind farm, and in the meantime, the wind turbine is not operational. On the other hand, components with similar failure rates, such as frequency converters, contribute much less to the downtime since spare parts are brought to site by faster by workboat vessels. In addition, the limited number of spare parts in stock is also a cause for downtime since more spare parts may be necessary for repair than available at stock, thus creating a spare waiting time. The spares problem is addressed in §4.3
Fig. 4: Components Contribution to total failure rate Etrecheck 4 1 – for troubleshooting your macros.
Fig. 5: Components Contribution to total downtime
The resulting WT availability is 80%, and the energy produced by the entire wind farm is 763697 MWh/year at a cost of 23.4 MЄ (O&M only). The resulting cost of energy is 31Є/MWh produced, and the capacity factor of the wind farm is 36.3%. See Scenario 1 in Table 2.
4. Optimization Scenarios
4.1 Inspections Optimization
The inspection schedule of the baseline scenario gives an identical weight to each WT component. While this approach is not unusual in existing installations, it is somewhat unjustified. An adapted inspection schedule should be able to improve the system availability by accounting for the different reliability rates and degradation process. The Inspection Optimization module of the apmOptimizer is used for performing the optimization, with the optimization objective being the minimization of total costs, i.e. maintenance costs, failure damage costs and downtime costs (revenue losses). Optionally, it is possible to define minimal required availability or reliability at component or system level. Since inspections are planned in advance, required spare parts can also be ordered in advance and provided on time.
The optimization results in the optimal inspections schedule shown in Table 3:
Table 3: Optimal Inspection Schedule
The interval unit is weeks, such that, for example, the optimization recommends performing inspection on the yaw system every 30 weeks. The user is free to accept or reject these recommendations, according to judgment. When possible, inspections are grouped in order to reduce cost. After accepting the recommendations, the resulting RAM/LCC and production parameters are computed, see scenario 2 in Table 2. Optimization yields very significant results: The WT availability increases by 7.3 points, which translates in a 9% increase in energy production. From the cost perspective, the effect is even more evident through a 26.5% reduction in corrective maintenance costs, as well as a reduction in preventive maintenance costs, due to better oriented inspections, although many more inspections are performed (inspections costs vary according to equipment). Overall, the cost of produced energy is reduced by about 30%.
The advantage of performing optimization by using an optimization algorithm, versus Monte Carlo type simulations, is clearly seen by looking at the proposed inspections schedule. The optimization algorithm is powerful enough to yield original solutions that provide the strongest cost reductions and performance improvements.
4.2 Condition Monitoring System Scenario – Preventive Maintenance Optimization
The effectiveness of implementing a CMS system is investigated here. CMS systems are useful in the case of offshore farms since they provide operator failure detection and identification, and enable scheduling a repair/replacement with much more precision. However, these systems are still expensive and do not always offer a clear view of what is really happening in the turbine.
In the present scenario, we assume that a CMS is installed on the following items: Gearbox, pitch system and yaw system (the main downtime drivers) and that their degradation is continuously measured. Coupled with an assumed prediction model, they enable predicting the time-to-failure (TTF) density distribution at a given time, and then decide on an optimal maintenance planning method that best accounts for maintenance costs and other maintenance tasks.
In our model, we assume that the monitored equipment now has a normally distributed failure time (instead of exponential) with an unchanged mean value, thus reflecting the results of the condition monitoring and prediction analysis. The previously defined inspections for the 3 items are cancelled and the Preventive Maintenance Optimization module is now used to find the best schedule to perform preventive replacement. Again, the optimization objective is a minimization of the total lifecycle costs. Inspection optimization is then updated to adapt the new maintenance schedule. The preventive replacement and updated inspections schedule recommended by the apmOptimizer are shown in Tables 4 and 5:
Table 4: Optimal Preventive Maintenance schedule (screenshot)
Table 5: Updated Inspections Schedule
The three systems are now subject to replacement only, and it can be observed that the inspection schedule has been adjusted to account for these replacements.
The resulting reliability, performance and cost metrics are summarized in scenario 3 of Table 2.
For the sake of comparison, an average investment cost of 1200 Є per CMS system (total CMS cost of 288000Є) has been included in the cost computations. Even with this additional cost, the implementation of CMS remains worthwhile and brings a reduction in O&M costs of more than 3.5MЄ per year compared to the previous scenario. However, this improvement has to be tempered by the fact that:
– Deployment of the CMS systems in the wind turbine could affect the turbine's reliability, since the CMS may fail as well.
-We assume that 100% of the degradations are clearly detected, although there are cases where signals cannot be clearly interpreted, or are not sufficient for drawing conclusions.
4.3 Spare part level optimization
Optimizing the inventory level of spare parts is an important stage in reducing downtime, especially for parts that have very long lead or turnaround time. After inspections and preventive maintenance have been optimized, the requirement for spares is modified and should be also optimized. For that purpose, we use the spares optimization module of the apmOptimizer. The optimization algorithm objective is to minimize the total costs and damage. The program provides the optimal initial spares quantity as well as the required spares that must be ordered during lifetime in order to replenish stocks of condemned parts.
As a result of the spare optimization, availability increases to 93.6%, as well as the energy produced. The reduction in corrective maintenance costs reflects a decrease in revenue losses due to downtime. The cost of energy produced decreases a little more to 14Є/MWh. See scenario 4 in Table 2.
5 Conclusions and future work
This case study has demonstrated the capabilities of apmOptimizer in efficiently modeling an offshore wind farm, and proposing original maintenance configurations to generate significant downtime and O&M cost reductions. Starting from a hypothetical yet representative baseline model, the inspection, preventive maintenance and spare parts modules have successively reduced the downtime and improved the total amount of energy produced. It has been shown that the knowledge of the failure modes of each component, and especially of the associated degradation process, enables defining the optimal inspection schedule and reducing the overall downtime.
The rationale behind using condition monitoring systems for early detection of the degradation process has been highlighted, and proven to be worth the investment. Moreover, the use of failure distributions extracted from on-site measurements was shown to be profitable for optimization of preventive replacements.
In a future case study, a more detailed model of the wind turbine components, as well as field data from real wind farms installations will be used to extract accurate reliability figures. This field data will be used to evaluate real failure distributions for all components instead of the usual assumptions.
6 References
[1] European wind Energy Association (EWEA) (2011), Wind in Our Sails.
[2] Jannie Sonderkar Nielsen, PhD (2013) Risk-Based Operation and Maintenance of Offshore Wind Turbines, Aalborg University.
[3] BQR Reliability Engineering Ltd, http://www.bqr.com
Onyx 3 2 2 – Maintenance And Optimization Tools
[4] ReliaWind Deliverable D 6.7 – (2010), Report
Recommendations from the ReliaWind Consortium for the Standardisation for the Wind Industry of Wind Turbine Reliability Taxonomy, Terminology and Data Collection
[5] Alexander Karyotakis, (2011) On the Optimisation of Operation and Maintenance Strategies for Offshore Wind Farms, Department of Mechanical Engineering University College London
Onyx 3 2 2 – Maintenance And Optimization Tool Software
[6] Reliawind Deliverable D.1.3 (2010), Report on Wind Turbine Reliability Profiles
[7] B. Maples, G. Saur, M. Hand, R. van de Pietermen and T. Obdam – NREL (2013), Installation, Operation, and Maintenance Strategies to Reduce the Cost of Offshore Wind Energy.
[8] Eugene C. Morgan, Matthew Lackner, Richard M. Vogel, Laurie G. Baise, (2011), Probability distributions for offshore wind speeds, Energy Conversion and Management Journal, No52, pp 15-26
[9] L.W.M.M. Rademakers, H. Braam, (2002), O&M Aspects of the 500MW Offshore Wind Farm at NL7, DOWEC project